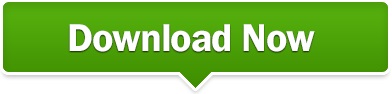
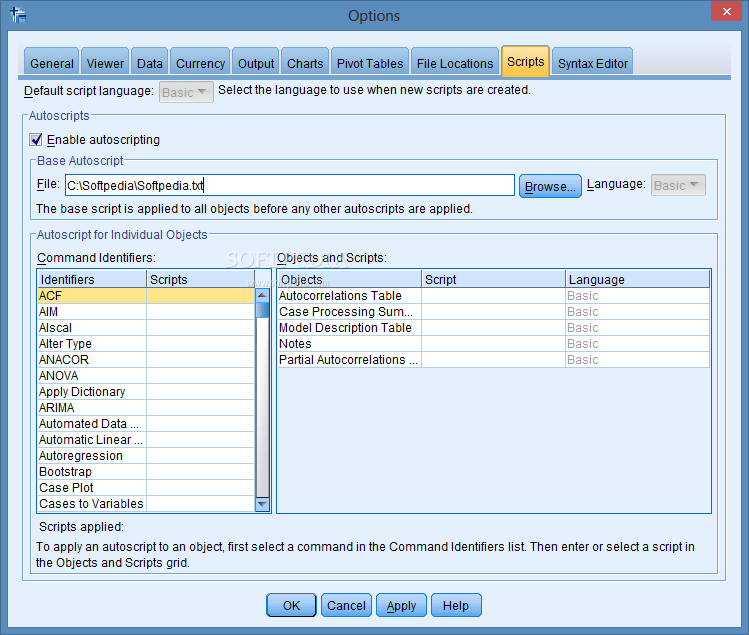
Therefore, intervention condition will be a Binomially distributed variable, this time with one trial (i.e., assignment to intervention or not) that has a 50% chance of being assigned to the intervention or control conditions. Participants have an equal chance of being assigned to the control or intervention conditions. Intervention condition is going to be a 2-level categorical variable that represents random assignment to a control condition or a mindfulness-based cognitive therapy intervention. Normally, we would define a standard deviation for a variable we are simulating, but physical stress is the dependent variable and the dependent variable’s variance will be set by the simulation process. I chose a mean value of 3.15 arbitrarily, but I generally guess that most people are below the midpoint (i.e., \(\mu 1\)). The values will be integers ranging between 1 - 7. With physical stress, I was imagining a single item, 1 - 7 Likert scale that people would use to rate, “Have you felt worn down physically from stress?” (1 = Not at All, 7 = Extremely). These choices are explained in further detail below Table 1. Otherwise, when creating the table below, I simply made up parameters that seemed plausible and reflected my expectations for each variable. If you already know those parameters (e.g., from existing data or published papers), then that would be a great source for good parameters. So, start by identifying the distribution that the variable should follow and then make up parameters for the distribution. You truly just make them up, but you want them to be plausible. When you simulate data, all the variable properties are made up by you. From the syntax above, we see that there are 3 variables needed for the analysis: DESIGN=condition centered_neuroticism condition*centered_neuroticismĢ.1. GLM physical_stress WITH condition centered_neuroticism That GLM syntax should look like this: * Planned GLM Syntax. In this example, physical stress ( \(physical\_stress_i\)) will be predicted from a variable representing random assignment to a control condition or intervention ( \(condition_i\)), neuroticism ( \(centered\_neuroticism_i\)), and their interaction. This example simulates data that could be analyzed with a GLM, specifically a moderated regression model. If you have trouble, then please let me know and hopefully I can debug it.ġ.

You should be able to open each file, select all, and run the syntax.
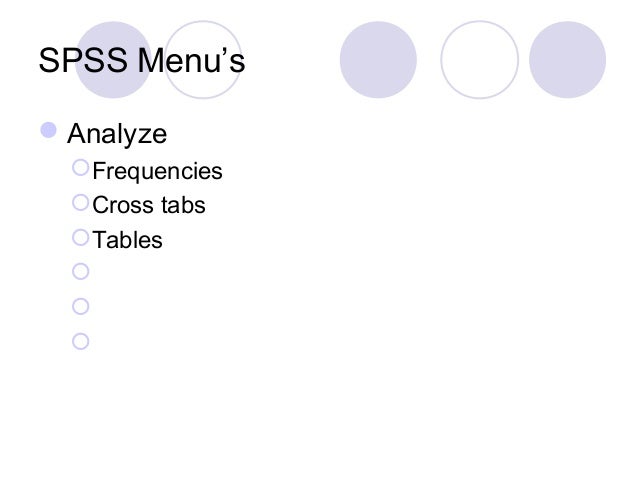
Simulation-Tutorial-Example-4-Syntax.sps.Simulation-Tutorial-Example-3-Syntax.sps.Simulation-Tutorial-Example-2-Syntax.sps.The last two examples assume you have familiarity with multilevel or structural equation modelling, but they are optional (i.e., you could stop reading after Example 2).Įxamples 2 through 4 each have accompanying syntax files linked below. The first two examples assume that you are familiar with the general linear model (GLM). The latter two examples extend the basic simulation tutorial to data that could be analyzed with a multilevel model (Example 3) or a structural equation model (Example 4). Therefore, both SPSS and this tutorial separates the process of data simulation into planning (Example 1) and data-generation steps (Example 2) at first, and then these steps are merged together in both Examples 3 and 4. That means you have to make a lot of decisions. Simulating data gives you power over every aspect of the data that result. I hope the concepts introduced in this tutorial serve as the building blocks you need to simulate the data you need. This tutorial introduces a number of basic concepts in data simulation using the statistical package, SPSS 26.0.
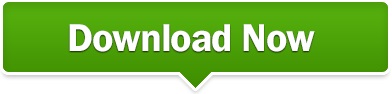